
Research at the Söding lab

High-throughput techniques are changing biological research
Experiments based on determining the sequences of DNA or RNA can now probe with unprecedented breadth and depth these mechanisms by which cells regulate the expression of genes into proteins. The rapid improvement of high-throughput sequencing technology in the last decade is thus boosting the pace of progress in biological research.
Sequencing technologies are also gaining increasing importance in medical research. In systems medicine, researchers aim to understand the origins of most common diseases by investigating what changes in the genomes of patients predispose to these diseases and what the mechanisms are by which these changes influence disease risk. These insights will help us to develop better drugs to prevent and treat common diseases.
A great advantage of the novel high-throughput, data-driven approach to biological research is that it is unbiased and can lead to unexpected discoveries, as it allows us to ask many questions to the data in little time without the need to formulate concrete hypotheses before the experiment is done.
But the data are often noisier than measurements from conventional, low throughput methods. Our group develops statistical and computational methods to make better use of the information hidden in these data. In this way we aim to facilitate data-driven approaches to cell and developmental biology, genetics, microbiology, and systems medicine.
Tools for sequence searching, protein function and structure prediction
Our group develops computational methods for predicting the structure, function, and evolution of proteins, the most important building blocks of cells. We develop statistical methods that enable us to make use of the vast amount of sequence information that is becoming available at an ever-increasing pace.
The goal is to provide life scientists with more and more powerful tools in order to guide their experimental work. Our software for the detection of remote common ancestry between proteins based on their sequences (HHpred, HH-suite) is widely used to predict the function and structure of proteins.

Our software MMseqs2 combines high sensitivity to detect related proteins with an extremely high search speed. MMseqs2 is particulary useful in metagenomics, a very dynamic research field that relies on sequencing samples directly from the environment. Due to the huge amounts of metagenomic and -transcriptomic sequencing data being generated, and because many of the sequences cannot be related to any known protein sequences, we need faster and also more sensitive search tools. We are developing a new paradigm of metagenomic sequence analysis based on analyzing not every metagenomic sample by itself but by intergrating the analysis of all samples from similar environments together. This is made possible by our new protein sequence clustering algorithm Linclust that can cluster sequences into homologous gropus (i.e. related by common descent) in a time linear instead of quadratic in the number of sequenes. This huge speed advantage opens up possibilities for new analysis approaches.
See: Quantum leap in fast and deep protein sequence similarity searching
Understanding the genome's “regulatory code”
It is one of the great enigmas of life how a single fertilized cell can develop into a complex, multicellular organism composed of hundreds of different types of cells. The organism’s genome directs the cells to follow individual molecular programs in which the expression of genes into proteins is switched on and off depending on the exact time and cell type.
We want to help in understanding how the most important level of the regulation of gene expression, namely transcriptional regulation, is encoded in each gene's regulatory regions. We develop computational methods to analyze these regions and to detect regulatory sequence motifs. We also want to predict transcription rates, using probabilistic modeling, statistical physics, and machine learning techniques. We collaborate extensively with experimental groups to elucidate the molecular processes regulating the various steps of transcription.
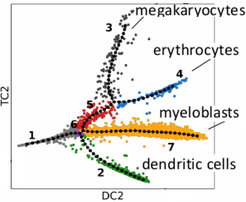
We develop statistical methods for the derivation of cellular lineage trees and to analyze the underlying gene regulatory networks that control these lineage commitment and differentiaition events, processes that are at the heart of understanding the molecular programs that the genome encodes. We make use of gene expression measurements of hundreds to thousands of single cells. This new field has the potential to revolutionize developmental biology by providing highly time-resolved time courses data of differentiating cells in their natural environments. By building models of the differentiation process we can perform model-based averaging over many cells and thus overcome the limits posed by the high noise levels in the gene expression levels of individual cells.
Systems medicine and precision medicine

Large amounts of valuable medical data are becoming available that will allow us as a community to better understand why and how common diseases such as coronary artery disease, Parkinson’s or Alzheimer’s disease originate, and to develop patient-specific, prophylactic and therapeutic treatments. Our group analyzes data with genotypes of hundreds of thousands of patients with and without various diseases. Using specifically developed statistical models, we learn the link between genetic variants and the disease risks. We also analyse and integrate data on how genetic variants influence the expression of genes in various tissues of the human body. We then build statistical models to integrate these datasets and predict groups of genes whose deregulated expression incereases the risk of developing a common disease.