MPI-Nat Seminar Series: Towards predicting gene expression from sequence
MPI-Nat Seminar Series
- Datum: 24.11.2022
- Uhrzeit: 13:00 - 14:00
- Vortragende(r): Jussi Taipale
- University of Cambridge, UK, Karolinska Institutet, Sweden, Univ. of Helsinki, Finland
- Ort: Max-Planck-Institut für Multidisziplinäre Naturwissenschaften (MPI-NAT, Faßberg-Campus)
- Raum: Ludwig Prandtl Hall
- Gastgeber: Patrick Cramer
- Kontakt: elisa.oberbeckmann@mpinat.mpg.de
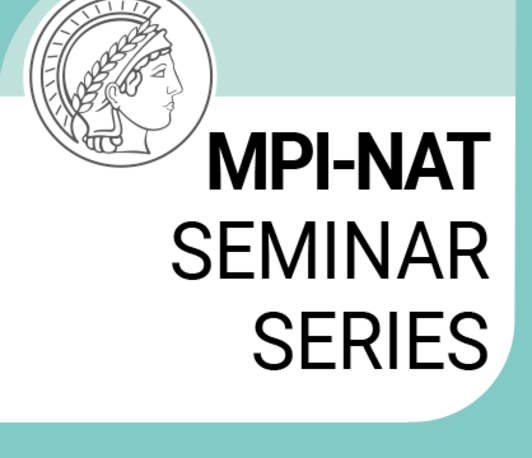
Understanding the information encoded in the human genome requires two genetic codes, the first code specifies how mRNA sequence is converted to protein sequence, and the second code determines when and where the mRNAs are expressed. Although the proteins that read the second, regulatory code – transcription factors (TFs) – have been largely identified, the code is poorly understood. In other words, we still cannot effectively predict when and where genes are expressed based on their DNA sequence. Our solution to this problem is the application of overwhelming experimental force combined with advanced computational methods. For this purpose, we have generated several genome-scale datasets, including sequence-specific binding affinities of human TFs to unmodified and epigenetically modified DNA. We have also begun to identify the major unknown factors in our quantitative understanding of transcription by performing several experiments that bridge the gap between in vivo analyses such as eQTLs, RNA-seq and ChIP-seq and in vitro studies such as SELEX. These approaches include analysis of TF binding to genomic variants, TF binding in the presence of the nucleosome, determining DNA-binding activities of all TFs from distinct cell types, and measuring transcriptional activities of TF motifs, genomic sequences and fully random DNA sequences in vivo. In particular, the random DNA experiments enable analysis of sequence-space that is several orders of magnitude larger than that of the human genome. We believe that application of machine-learning methods to such datasets will enable a full "predictive understanding" of gene expression: determining the rules that would be both understandable at a conceptual level by humans and sufficient for computational generation of accurate quantitative predictions.